Machine learning: market opportunities
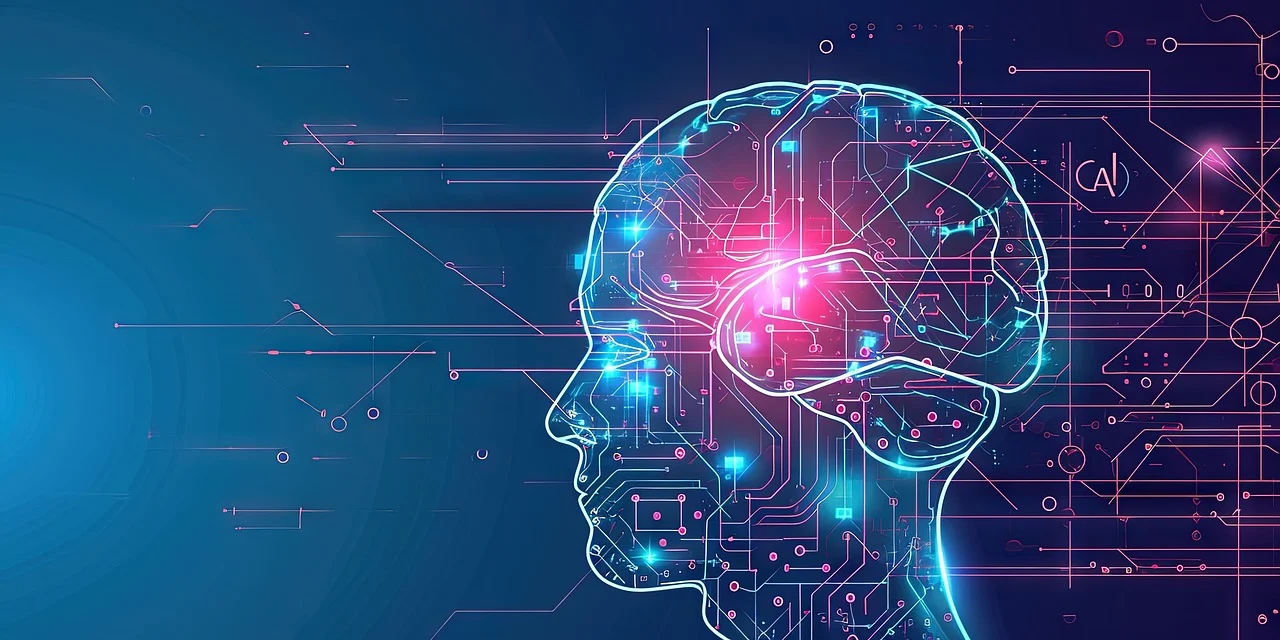
Fortune Business Insights estimates the potential market opportunity for machine learning at $26 billion, with compound annual growth rates of 36% through 2030.What is machine learning? A field of artificial intelligence that gives systems the ability to learn and improve from experience without being explicitly programmed. It involves computers and programs that can be programmed to operate with minimal human intervention and, in some cases, can learn and/or update themselves.Essentially, it focuses on the development of algorithms and models that allow computers to process large amounts of data, derive patterns from this data, and then make decisions or predictions based on it.In other words, this type of artificial intelligence makes it possible to perform specific tasks without being explicitly programmed to do so.Thus, it finds relationships between variables and outcomes, and discovers interactions between multiple variables.
Machine Learning
How much potential annual value could machine learning applications with fully fault-tolerant quantum computers generate? According to predictions by Boston Consulting Group, between $150 billion and $220 billion worldwide for end users and technology providers by 2050.Machine learning has three main subfields: supervised, unsupervised and reinforcement learning.
Supervised
Labeled data is used to learn important predictive features for some response variable. In this variant, models are trained using labeled data, i.e., data that has a known input and output. The model learns to make predictions or decisions based on training examples. An example: predicting the price of a house based on its characteristics.
Unsupervised
Here there are no labels, but rather observed characteristics (or features) of entities are used to group them into clusters. The objective is to discover underlying patterns or structures in the data. As an example, this is used in applications such as customer segmentation.
Reinforcement learning
In reinforcement learning, models learn to make sequential decisions by interacting with an environment and receiving feedback in the form of rewards or punishments. For example, it is used in applications such as strategy games and process optimization.